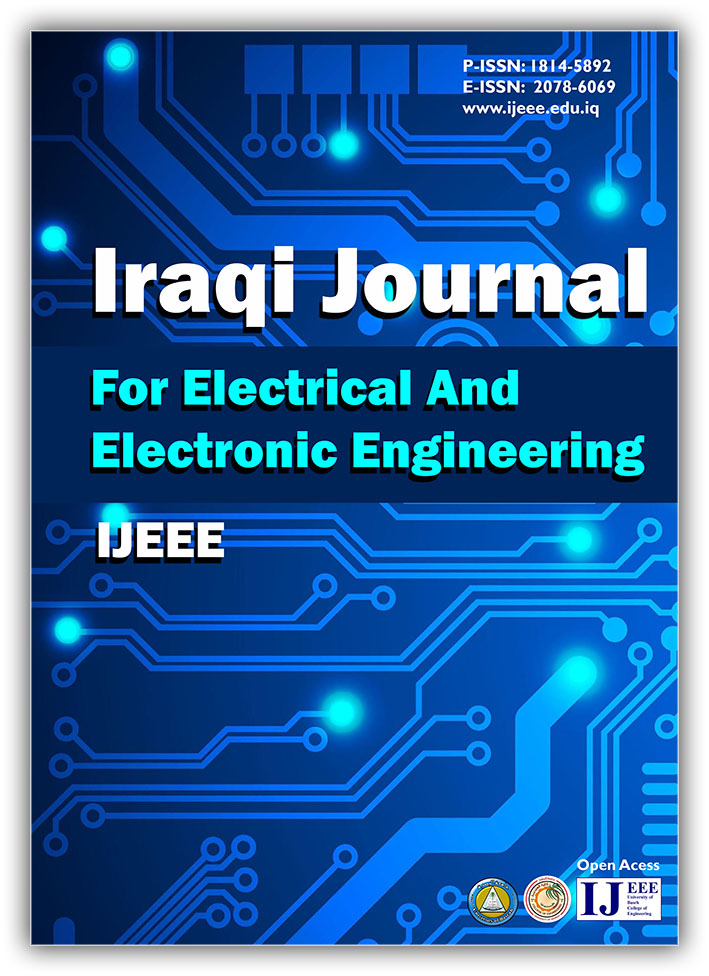
December 2024
<Previous Issue Next Issue>
Electronic Version
Open Access | |
A Secure Image Cryptographic Algorithm Based on Triple Incorporated Ciphering Stages | |
Sura F. Yousif, Abbas Salman Hameed, and Dheyaa T. Al-Zuhairi | |
Pages: 1-21 | |
Version of record online: 26 March 2024 Full Text (PDF) |
|
Lately, image encryption has stand out as a highly urgent demand to provide high security for digital images against use and unauthorized distribution. A lot of existing researches use chaotic systems, symmetric or asymmetric schemes for image encryption, but cryptosystem based on one encryption technique only, faces many challenges like weak security and low complexity. Therefore, incorporating two or more different ciphering methods yields a secure and efficient algorithm to protect image information. In this work, a new image cryptosystem is suggested by joining zigzag scan technique, RSA algorithm and chaotic systems. These three security factors introduce Triple Incorporated Ciphering stages system (TIC). Initially, the plaintext image is divided into $8\times 8$ non-overlapping blocks, then the odd blocks are isolated from the even blocks. After that, a new modified zigzag scan in two different directions is adopted for shuffling pixels in the odd and even blocks. This operation effectively enhances the shuffling degree. Next, the RSA algorithm is utilized after combining the scrambled blocks in one matrix. Finally, chaotic systems are implemented on the resultant encrypted matrix to complete the ciphering process. The chaos is implemented in two steps; confusion and diffusion. Duffing map is exploited in the confusion stage, whereas L”u system is adopted on the shuffled matrix in the diffusion stage. The simulation results show the superiority of TIC in both security and attacks robustness compared to other cryptographic algorithms. Therefore, TIC can be exploited in real-time communication systems for secure image transmission. |
|
Open Access | |
On the Actuation Technologies of Biomedical Microrobot: A Summarized Review | |
Anwar Hamza Bresam, and Haider Al-Mumen | |
Pages: 22-32 | |
Version of record online: 23 April 2024 Full Text (PDF) |
|
In recent years, wireless microrobots have gotten more attention due to their huge potential in the biomedical field, especially drug delivery. Microrobots have several benefits, including small size, low weight, sensitivity, and flexibility. These characteristics have led to microscale improvements in control systems and power delivery with the development of submillimeter-sized robots. Wireless control of individual mobile microrobots has been achieved using a variety of propulsion systems, and improving the actuation and navigation of microrobots will have a significant impact. On the other hand, actuation tools must be integrated and compatible with the human body to drive these untethered microrobots along predefined paths inside biological environments. This study investigated key microrobot components, including medical applications, actuation systems, control systems, and design schemes. The efficiency of a microrobot is impacted by many factors, including the material, structure, and environment of the microrobot. Furthermore, integrating a hybrid actuation system and multimodal imaging can increase the microrobot’s navigation effect, imaging algorithms, and working environment. In addition, taking into account the human body’s moving distance, autonomous actuating technology could be used to deliver microrobots precisely and quickly to a specific position using a combination of quick approaches. |
|
Open Access | |
Taguchi Method Based Node Performance Analysis of Generous TIT- for-TAT Cooperation of AD-HOC Networks | |
Noor Kareem Jumaa, Auday A.H. Mohamad, Abbas Muhammed Allawy, and Ali A. Mohammed | |
Pages: 33-44 | |
Version of record online: 24 April 2024 Full Text (PDF) |
|
Ad-Hoc networks have an adaptive architecture, temporarily configured to provide communication between wireless devices that provide network nodes. Forwarding packets from the source node to the remote destination node may require intermediate cooperative nodes (relay nodes), which may act selfishly because they are power-constrained. The nodes should exhibit cooperation even when faced with occasional selfish or non-cooperative behaviour from other nodes. Several factors affect the behaviour of nodes; those factors are the number of packets required to redirect, power consumption per node, and power constraints per node. Power constraints per node and grade of generosity. This article is based on a dynamic collaboration strategy, specifically the Generous Tit-for-Tat (GTFT), and it aims to represent an Ad-Hoc network operating with the Generous Tit-for-Tat (GTFT) cooperation strategy, measure statistics for the data, and then analyze these statistics using the Taguchi method. The transfer speed and relay node performance both have an impact on the factors that shape the network conditions and are subject to analysis using the Taguchi Method (TM). The analyzed parameters are node throughput, the amount of relay requested packets produced by a node per number of relays requested packets taken by a node, and the amount of accepted relay requested by a node per amount of relay requested by a node. A Taguchi L9 orthogonal array was used to analyze node behaviour, and the results show that the effect parameters were number of packets, power consumption, power constraint of the node, and grade of generosity. The tested parameters influence node cooperation in the following sequence: number of packets required to redirect (N) (effects on behaviour with a percent of 6.8491), power consumption per node (C) (effects on behaviour with a percent of 0.7467), power constraints per node (P) (effects on behaviour with a percent of 0.6831), and grade of generosity (ε) (effects on behaviour with a percent of 0.4530). Taguchi experiments proved that the grade of generosity (GoG) is not the influencing factor where the highest productivity level is, while the number of packets per second required to redirect also has an impact on node behaviour. |
|
Open Access | |
An Adaptive Steganography Insertion Technique Based on Cosine Transform | |
Taif Alobaidi, and Wasfy Mikhael | |
Pages: 45-58 | |
Version of record online: 28 April 2024 Full Text (PDF) |
|
In the last couple decades, several successful steganography approaches have been proposed. Least Significant Bit (LSB) Insertion technique has been deployed due to its simplicity in implementation and reasonable payload capacity. The most important design parameter in LSB techniques is the embedding location selection criterion. In this work, LSB insertion technique is proposed which is based on selecting the embedding locations depending on the weights of coefficients in Cosine domain (2D DCT). The cover image is transformed to the Cosine domain (by 2D DCT) and predefined number of coefficients are selected to embed the secret message (which is in the binary form). Those weights are the outputs of an adaptive algorithm that analyses the cover image in two domains (Haar and Cosine). Coefficients, in the Cosine transform domain, with small weights are selected. The proposed approach is tested with samples from the BOSSbase, and a custom-built databases. Two metrics are utilized to show the effectiveness of the technique, namely, Root Mean Squared Error (RMSE), and Peak Signal-to-Noise Ratio (PSNR). In addition, human visual inspection of the result image is also considered. As shown in the results, the proposed approach performs better, in terms of (RMSE, and PSNR) than commonly employed truncation and energy based methods. |
|
Open Access | |
Design and Implementation of PID Controller for the Cooling Tower’s pH Regulation Based on Particle Swarm Optimization PSO Algorithm | |
Basim Al-Najari, Assoc. Prof. Dr. Chong Kok Hen, Prof. Dr. Johnny Koh Siaw Paw, and Prof. Dr. Ali Fadhil Marhoon | |
Pages: 59-67 | |
Version of record online: 30 April 2024 Full Text (PDF) |
|
The PH regulation of cooling tower plant in southern fertilizers company (SCF) in Iraq is important for industry pipes protection and process continuity. According to the Mitsubishi standard, the PH of cooling water must be around (7.1 to 7.8). The deviation in PH parameter affects the pipes, such as corrosion and scale. Acidic water causes pipes to corrode, and alkaline water causes pipes to scale. The sulfuric acid solution is used for PH neutralization. The problem is that the sulfuric acid is pumped manually in the cooling tower plant every two or three hours for PH regulation. The manual operation of the sulfuric acid pump makes deviations in the PH parameter. It is very difficult to control the PH manually. To solve this problem, a PID controller for PH regulation was used. The reason for using the PID controller is that the PH response is irregular through the neutralization process. The methodology is to calculate the transfer function of the PH loop using the system identification toolbox of MATLAB, to design and implement a PID controller, to optimize the PID controller response using particle swarm optimization PSO algorithm, and to make a comparison among several tuning methods such as Ziegler Nichols (ZN) tuning method, MATLAB tuner method, and PSO algorithm tuning method. The results showed that the PSO-based PID controller tuning gives a better overshoot, less rise time, and an endurable settling time than the other tuning methods. Hence, the PH response became according to the target range. The experimental results showed that the PH regulation improved using the PSO-based PID controller tuning. |
|
Open Access | |
Design a Power System of 1760W Based on a Twin Inverter and a Fuzzy Logic Controller | |
Samhar Saeed Shukir | |
Pages: 68-76 | |
Version of record online: 9 June 2024 Full Text (PDF) |
|
The increasing demand for electricity due to population expansion has led to frequent interruptions in electrical power, so there are backup power lines everywhere, especially in the sectors of education, health, banking, transportation and communications. DC sources are beginning to become widely spread in terms of low maintenance requirements, no need for refueling, and no pollutant emission in these institutions. The problems of DC systems are; losses in DC system components, and change in output voltage as loads change. This research presents a power system that generates 1760W AC power from batteries bank, the system consists of a twin inverter to reduce losses in switches and filters, and thus improving the efficiency and the power factor of the system, and fuzzy logic controllers to regulate the output voltage of the converter and inverter. Modeling and simulation in MATLAB / Simulink showed obtaining a constant load voltage with acceptable values of total harmonics distortion (THD) under different conditions of loads and batteries. |
|
Open Access | |
Handwritten Signature Verification Method Using Convolutional Neural Network | |
Wijdan Yassen A. AlKarem, Eman Thabet Khalid, and Khawla. H. Ali | |
Pages: 77-84 | |
Version of record online: 9 June 2024 Full Text (PDF) |
|
Automatic signature verification methods play a significant role in providing a secure and authenticated handwritten signature in many applications, to prevent forgery problems, specifically institutions of finance, and transections of legal papers, etc. There are two types of handwritten signature verification methods: online verification (dynamic) and offline verification (static) methods. Besides, signature verification approaches can be categorized into two styles: writer dependent (WD), and writer independent (WI) styles. Offline signature verification methods demands a high representation features for the signature image. However, lots of studies have been proposed for WI offline signature verification. Yet, there is necessity to improve the overall accuracy measurements. Therefore, a proved solution in this paper is depended on deep learning via convolutional neural network (CNN) for signature verification and optimize the overall accuracy measurements. The introduced model is trained on English signature dataset. For model evaluation, the deployed model is utilized to make predictions on new data of Arabic signature dataset to classify whether the signature is real or forged. The overall obtained accuracy is 95.36% based on validation dataset. |
|
Open Access | |
Study of Chaotic-based Audio Encryption Algorithms: A Review | |
Alaa Shumran and Abdul-Basset A. Al-Hussein | |
Pages: 85-103 | |
Version of record online: 11 June 2024 Full Text (PDF) |
|
Nowadays, multimedia communication has become very widespread and this requires it to be protected from attackers and transmitted securely for reliability. Encryption and decryption techniques are useful in providing effective security for speech signals to ensure that these signals are transmitted with secure data and prevent third parties or the public from reading private messages. Due to the rapid improvement in digital communications over the recent period up to the present, the security of voice data transmitted over various networks has been classified as a favored field of study in earlier years. The contributions to audio encryption are discussed in this review. This Comprehensive review mainly focuses on presenting several kinds of methods for audio encryption and decryption the analysis of these methods with their advantages and disadvantages have been investigated thoroughly. It will be classified into encryption based on traditional methods and encryption based on advanced chaotic systems. They are divided into two types, continuous-time system, and discrete-time system, and also classified based on the synchronization method and the implementation method. In the fields of information and communications security, system designers face many challenges in both cost, performance, and architecture design, Field Programmable gate arrays (FPGAs) provide an excellent balance between computational power and processing flexibility. In addition, encryption methods will be classified based on Chaos-based Pseudo Random Bit Generator, Fractional-order systems, and hybrid chaotic generator systems, which is an advantageous point for this review compared with previous ones. Audio algorithms are presented, discussed, and compared, highlighting important advantages and disadvantages. Audio signals have a large volume and a strong correlation between data samples. Therefore, if traditional cryptography systems are used to encrypt such huge data, they gain significant overhead. Standard symmetric encryption systems also have a small key-space, which makes them vulnerable to attacks. On the other hand, encryption by asymmetric algorithms is not ideal due to low processing speed and complexity. Therefore, great importance has been given to using chaotic theory to encode audio files. Therefore, when proposing an appropriate encryption method to ensure a high degree of security, the key space, which is the critical part of every encryption system, and the key sensitivity must be taken into account. The key sensitivity is related to the initial values and control variables of the chaotic system chosen as the audio encryption algorithm. In addition, the proposed algorithm should eliminate the problems of periodic windows, such as limited chaotic range and non-uniform distribution, and the quality of the recovered audio signal remains good, which confirms the convenience, reliability, and high security. |
|
Open Access | |
An Experimental Investigation on VSI-fed Induction Motor using Xilinx ZYNQ-7000 SoC Controller | |
Santosh Yadav Maddu, and Dr. Nitin Ramesh Bhasme | |
Pages: 104-114 | |
Version of record online: 19 June 2024 Full Text (PDF) |
|
In medium voltage and high-power drive applications, pulse width modulation (PWM) techniques are widely used to achieve effective speed control of AC motors. In real-time, an industrial drive system requires reduced hardware complexity and low computation time. The reliability of the AC drive can be improved with the FPGA (field programmable gate array) hardware equipped with digital controllers. To improve the performance of AC drives, a new FPGA-based Wavect real-time prototype controller (Xilinx ZYNQ-7000 SoC) is used to verify the effectiveness of the controller. These advanced controllers are capable of reducing computation time and enhancing the drive performance in real-time applications. The comparative performance analysis is carried out for the most commonly used voltage source inverter (VSI)-based PWM techniques such as sinusoidal pulse width modulation (SPWM) and space vector pulse width modulation (SVPWM) for three-phase, two-level inverters. The comparative study shows the SVPWM technique utilizes DC bus voltage more effectively and produces less harmonic distortion in terms of higher output voltage, flexible control of output frequency, and reduced harmonic distortion at output voltage for motor control applications. The simulation and hardware results are verified and validated by using MATLAB/Simulink software and FPGA-based Wavect real-time controller respectively. |
|
Open Access | |
Epileptic detection based on deep learning: A review | |
Ola M. Assim and Ahlam F. Mahmood | |
Pages: 115-126 | |
Version of record online: 19 June 2024 Full Text (PDF) |
|
Epilepsy, a neurological disorder characterized by recurring seizures, necessitates early and precise detection for effective management. Deep learning techniques have emerged as powerful tools for analyzing complex medical data, specifically electroencephalogram (EEG) signals, advancing epileptic detection. This review comprehensively presents cutting-edge methodologies in deep learning-based epileptic detection systems. Beginning with an overview of epilepsy’s fundamental concepts and their implications for individuals and healthcare are present. This review then delves into deep learning principles and their application in processing EEG signals. Diverse research papers to know the architectures—convolutional neural networks, recurrent neural networks, and hybrid models—are investigated, emphasizing their strengths and limitations in detecting epilepsy. Preprocessing techniques for improving EEG data quality and reliability, such as noise reduction, artifact removal, and feature extraction, are discussed. Present performance evaluation metrics in epileptic detection, such as accuracy, sensitivity, specificity, and area under the curve, are provided. This review anticipates future directions by highlighting challenges such as dataset size and diversity, model interpretability, and integration with clinical decision support systems. Finally, this review demonstrates how deep learning can improve the precision, efficiency, and accessibility of early epileptic diagnosis. This advancement allows for more timely interventions and personalized treatment plans, potentially revolutionizing epilepsy management. |
|
Open Access | |
A Dataset for Kinship Estimation from Image of Hand Using Machine Learning | |
Sarah Ibrahim Fathi and Mazin H. Aziz | |
Pages: 127-136 | |
Version of record online: 19 June 2024 Full Text (PDF) |
|
Kinship (Familial relationships) detection is crucial in many fields and has applications in biometric security, adoption, forensic investigations, and more. It is also essential during wars and natural disasters like earthquakes since it may aid in reunion, missing person searches, establishing emergency contacts, and providing psychological support. The most common method of determining kinship is DNA analysis which is highly accurate. Another approach, which is noninvasive, uses facial photos with computer vision and machine learning algorithms for kinship estimation. Each part of the Human -body has its own embedded information that can be extracted and adopted for identification, verification, or classification of that person. Kinship recognition is based on finding traits that are shared by every family. We investigate the use of hand geometry for kinship detection, which is a new approach. Because of the available hand image Datasets do not contain kinship ground truth; therefore, we created our own dataset. This paper describes the tools, methodology, and details of the collected MKH, which stands for the Mosul Kinship Hand, images dataset. The images of MKH dataset were collected using a mobile phone camera with a suitable setup and consisted of 648 images for 81 individuals from 14 families (8 hand situations per person). This paper also presents the use of this dataset in kinship prediction using machine learning. Google MdiaPipe was used for hand detection, segmentation, and geometrical key points finding. Handcraft feature extraction was used to extract 43 distinctive geometrical features from each image. A neural network classifier was designed and trained to predict kinship, yielding about 93% prediction accuracy. The results of this novel approach demonstrated that the hand possesses biometric characteristics that may be used to establish kinship, and that the suggested method is a promising way as a kinship indicator. |
|
Open Access | |
Medical Communication Systems Utilizing Optical Nanoantenna and Microstrip Technology | |
Munaf Fathi Badr, Ibrahim A. Murdas, and Ahmed Aldhahab | |
Pages: 137-153 | |
Version of record online: 26 June 2024 Full Text (PDF) |
|
Many technical approaches were implemented in the antenna manufacturing process to maintain the desired miniaturization of the size of the antenna model which can be employed in various applied systems such as medical communication systems. Furthermore, over the past several years, nanotechnology science has rapidly grown in a wide variety of applications, which has given rise to novel ideas in the design of antennas based on nanoscale merits, leading to the use of antennae as an essential linkage between the human body and the different apparatus of the medical communication system. Some medical applications dealt with different antenna configurations, such as microstrip patch antenna or optical nanoantenna in conjugate with sensing elements, controlling units, and monitoring instruments to maintain a specified healthcare system. This study summarizes and presents a brief review of the recent applications of antennas in different medical communication systems involving highlights, and drawbacks with explores recommended issues related to using antennas in medical treatment. |
|
Open Access | |
Advancements and Challenges in Hand Gesture Recognition: A Comprehensive Review | |
Bothina Kareem Murad, and Abbas H. Hassin Alasadi | |
Pages: 154-164 | |
Version of record online: 3 July 2024 Full Text (PDF) |
|
Hand gesture recognition is a quickly developing field with many uses in human-computer interaction, sign language recognition, virtual reality, gaming, and robotics. This paper reviews different ways to model hands, such as vision-based, sensor-based, and data glove-based techniques. It emphasizes the importance of accurate hand modeling and feature extraction for capturing and analyzing gestures. Key features like motion, depth, color, shape, and pixel values and their relevance in gesture recognition are discussed. Challenges faced in hand gesture recognition include lighting variations, complex backgrounds, noise, and real-time performance. |
|
Open Access | |
Design of High-Secure Digital/Optical Double Color Image Encryption Assisted by 9D Chaos and DnCNN | |
Rusul Abdulridha Muttashar, and Raad Sami Fyath | |
Pages: 165-181 | |
Version of record online: 3 July 2024 Full Text (PDF) |
|
With the rapid development of multimedia technology, securing the transfer of images becomes an urgent matter. Therefore, designing a high-speed/secure system for color images is a real challenge. A nine-dimensional (9D) chaotic-based digital/optical encryption schem is proposed for double-color images in this paper. The scheme consists of cascaded digital and optical encryption parts. The nine chaotic sequences are grouped into three sets, where each set is responsible for encryption one of the RGB channels independently. One of them controls the fusion, XOR operation, and scrambling-based digital part. The other two sets are used for controlling the optical part by constructing two independent chaotic phase masks in the optical Fourier transforms domain. A denoising convolution neural network (DnCNN) is designed to enhance the robustness of the decrypted images against the Gaussian noise. The simulation results prove the robustness of the proposed scheme as the entropy factor reaches an average of 7.997 for the encrypted color lena-baboon images with an infinite peak signal-to-noise ratio (PSNR) for the decrypted images. The designed DnCNN operates efficiently with the proposed encryption scheme as it enhances the performance against the Gaussian noise, where the PSNR of the decrypted Lena image is enhanced from 27.01 dB to 32.56 dB after applying the DnCNN. |
|
Open Access | |
Design Efficient Vedic-Multiplier for Floating-Point MAC Module | |
Fatima Tariq Hussein, and Fatemah K. AL-Assfor | |
Pages: 182-189 | |
Version of record online: 14 July 2024 Full Text (PDF) |
|
Multiplication-accumulation (MAC) operation plays a crucial role in digital signal processing (DSP) applications, such as image convolution and filters, especially when performed on floating-point numbers to achieve high-level of accuracy. The performance of MAC module highly relies upon the performance of the multiplier utilized. This work offers a distinctive and efficient floating-point Vedic multiplier (VM) called adjusted-VM (AVM) to be utilized in MAC module to meet modern DSP demands. The proposed AVM is based on Urdhva-Tiryakbhyam-Sutra (UT-Sutra) approach and utilizes an enhanced design for the Brent-Kung carry-select adder (EBK-CSLA) to generate the final product. A (6*6)-bit AVM is designed first, then, it is extended to design (12*12)-bit AVM which in turns, utilized to design (24*24)-bit AVM. Moreover, the pipelining concept is used to optimize the speed of the offered (24*24)-bit AVM design. The proposed (24*24)-bit AVM can be used to achieve efficient multiplication for mantissa part in binary single-precision (BSP) floating-point MAC module. The proposed AVM architectures are modeled in VHDL, simulated, and synthesized by Xilinx-ISE14.7 tool using several FPGA families. The implementation results demonstrated a noticeable reduction in delay and area occupation by 33.16% and 42.42%, respectively compared with the most recent existing unpipelined design, and a reduction in delay of 44.78% compared with the existing pipelined design. |
|
Open Access | |
A New Algorithm Based on Pitting Corrosion for Engineering Design Optimization Problems | |
Hussien A. Al-mtory, Falih M. Alnahwi, and Ramzy S. Ali | |
Pages: 190-206 | |
Version of record online: 27 July 2024 Full Text (PDF) |
|
This paper presents a new optimization algorithm called corrosion diffusion optimization algorithm (CDOA). The proposed algorithm is based on the diffusion behavior of the pitting corrosion on the metal surface. CDOA utilizes the oxidation and reduction electrochemical reductions as well as the mathematical model of Gibbs free energy in its searching for the optimal solution of a certain problem. Unlike other algorithms, CDOA has the advantage of dispensing any parameter that need to be set for improving the convergence toward the optimal solution. The superiority of the proposed algorithm over the others is highlighted by applying them on some unimodal and multimodal benchmark functions. The results show that CDOA has better performance than the other algorithms in solving the unimodal equations regardless the dimension of the variable. On the other hand, CDOA provides the best multimodal optimization solution for dimensions less than or equal to (5, 10, 15, up to 20) but it fails in solving this type of equations for variable dimensions larger than 20. Moreover, the algorithm is also applied on two engineering application problems, namely the PID controller and the cantilever beam to accentuate its high performance in solving the engineering problems. The proposed algorithm results in minimized values for the settling time, rise time, and overshoot for the PID controller. Where the rise time, settling time, and maximum overshoot are reduced in the second order system to 0.0099, 0.0175 and 0.005 sec., in the fourth order system to 0.0129, 0.0129 and 0 sec, in the fifth order system to 0.2339, 0.7756 and 0, in the fourth system which contains time delays to 1.5683, 2.7102 and 1.80 E-4 sec., and in the simple mass-damper system to 0.403, 0.628 and 0 sec., respectively. |
|
Open Access | |
Comparative Long-Term Electricity Forecasting Analysis: A Case Study of Load Dispatch Centres in India | |
Saikat Gochhait, Deepak K. Sharma, and Mrinal Bachute | |
Pages: 207-219 | |
Version of record online: 27 July 2024 Full Text (PDF) |
|
Accurate long-term load forecasting (LTLF) is crucial for smart grid operations, but existing CNN-based methods face challenges in extracting essential features from electricity load data, resulting in diminished forecasting performance. To overcome this limitation, we propose a novel ensemble model that integrates a feature extraction module, densely connected residual block (DCRB), long short-term memory layer (LSTM), and ensemble thinking. The feature extraction module captures the randomness and trends in climate data, enhancing the accuracy of load data analysis. |
|
Open Access | |
Analysis of Permanent Magnet Material Influence on Eddy Current Braking Efficiency | |
Ahmed M. Salman, Jamal A.-K. Mohammed, and Farag M. Mohammed | |
Pages: 220-225 | |
Version of record online: 27 July 2024 Full Text (PDF) |
|
Traditional friction brakes can generate problems such as high braking temperature and pressure, cracking, and wear, leading to braking failure and user damage. Eddy current brake systems (contactless magnetic brakes) are one method used in motion applications. They are wear-free, less temperature-sensitive, quick, easy, and less susceptible to wheel lock, resulting in less brake failure due to the absence of physical contact between the magnet and disc. Important factors that can affect the performance of the braking system are the type of materials manufactured for the permanent magnets. This paper examines the performance of the permanent magnetic eddy current braking (PMECB) system. Different kinds of permanent magnets are proposed in this system to create eddy currents, which provide braking for the braking system is simulated using FEA software to demonstrate the efficiency of braking in terms of force production, energy dissipation, and overall performance findings demonstrated that permanent magnets consisting of neodymium, iron, and boron consistently provided the maximum braking effectiveness. The lowest efficiency is found in ferrite, which has the second-lowest efficiency behind samarium cobalt. This is because ferrite has a weaker magnetic field. Because of this, the PMECBS based on NdFeB magnets has higher power dissipation values, particularly at higher speeds. |
|
Open Access | |
Design of Optimal STSMC Method Based on FPGA to Track the Trajectory of 2-DOF Robot Manipulator | |
Atheel K. Abdul Zahra and Wasan A. Wali | |
Pages: 226-235 | |
Version of record online: 2 August 2024 Full Text (PDF) |
|
This article emphasizes on a strategy to design a Super Twisting Sliding Mode Control (STSMC) method. The proposed controller depends on the device of Field Programmable Gate Array (FPGA) for controlling the trajectory of robot manipulator. The gains of the suggested controller are optimized using Chaotic Particle Swarm Optimization (PSO) in MATLAB toolbox software and Simulink environment. Since the control systems speed has an influence on their stability requirements and performance, (FPGA) device is taken in consideration. The proposed control method based on FPGA is implemented using Xilinx block sets in the Simulink. Integrated Software Environment (ISE 14.7) and System Generator are employed to create the file of Bitstream which can be downloaded in the device of FPGA. The results show that the designed controller based of on the FPGA by using System Generator is completely verified the effectiveness of controlling the path tracking of the manipulator and high speed. Simulation results explain that the percentage improvement in the Means Square Error (MSEs) of using the STSMC based FPGA and tuned via Chaotic PSO when compared with the same proposed controller tuned with classical PSO are 17.32 % and 13.98 % for two different cases of trajectories respectively. |
|
Open Access | |
The Effect of Quantum Dots on the Performance of the Solar Cell | |
Iman Mohsen Ahmed, Omar Ibrahim Alsaif, and Qais Th. Algwari | |
Pages: 236-242 | |
Version of record online: 4 August 2024 Full Text (PDF) |
|
Quantum dot solar cells are currently the subject of research in the fields of renewable energy, photovoltaics and optoelectronics, due to their advantages which enables them to overcome the limitations of traditional solar cells. The inability of ordinary solar cells to generate charge carriers, which is prevents them from contributing to generate the current in solar cells. This work focuses on modeling and simulating of Quantum Dot Solar Cells based on InAs/GaAs as well as regular type of GaAs p-i-n solar cells and to study the effect of increasing quantum dots layers at the performance of the solar cell. The low energy of the fell photons considers as one of the most difficult problems that must deal with. According to simulation data, the power conversion efficiency increases from (12.515% to 30.94%), current density rises from 16.4047 mA/cm2 for standard solar cell to 39.4775 mA/cm2) using quantum dot techniques (20-layers) compared to traditional type of GaAs solar cell. Additionally, low energy photons’ absorption range edge expanded from (400 to 900 nm) for quantum technique. The results have been modeled and simulated using (SILVACO Software), which proved the power conversion efficiency of InAs/GaAs quantum dot solar cells is significantly higher than traditional (p-i-n) type about (247%). |
|
Open Access | |
Efficient Path Planning in Medical Environments: Integrating Genetic Algorithm and Probabilistic Roadmap (GA-PRM) for Autonomous Robotics | |
Sarah Sabeeh, and Israa S. Al-Furati | |
Pages: 243-258 | |
Version of record online: 12 August 2024 Full Text (PDF) |
|
Path-planning is a crucial part of robotics, helping robots move through challenging places all by themselves. In this paper, we introduce an innovative approach to robot path-planning, a crucial aspect of robotics. This technique combines the power of Genetic Algorithm (GA) and Probabilistic Roadmap (PRM) to enhance efficiency and reliability. Our method takes into account challenges caused by moving obstacles, making it skilled at navigating complex environments. Through merging GA’s exploration abilities with PRM’s global planning strengths, our GA-PRM algorithm improves computational efficiency and finds optimal paths. To validate our approach, we conducted rigorous evaluations against well-known algorithms including A*, RRT, Genetic Algorithm, and PRM in simulated environments. The results were remarkable, with our GA-PRM algorithm outperforming existing methods, achieving an average path length of 25.6235 units and an average computational time of 0.6881 seconds, demonstrating its speed and effectiveness. Additionally, the paths generated were notably smoother, with an average value of 0.3133. These findings highlight the potential of the GA-PRM algorithm in real-world applications, especially in crucial sectors like healthcare, where efficient path-planning is essential. This research contributes significantly to the field of path-planning and offers valuable insights for the future design of autonomous robotic systems. |
|
Open Access | |
Ultra-Wide Band Printed Microstrip Patch Antenna with Two Band Rejection Feature | |
Asmaa H. Majeed | |
Pages: 259-265 | |
Version of record online: 12 August 2024 Full Text (PDF) |
|
This work presents a new design idea for a UWB printed micro strip patch antenna with two band-rejection features. The patch has an elliptical shape and its feeding using micro strip feeding line. To achieve the UWB, an elliptical slot was etched on a ground plane. The rejection of two-band is achieved with the addition of two different slots on the radiating patch, the first slot is inverted U shaped slot and the other is U-shaped slot, so there is no need for antenna’s additional size. The radiation pattern of the suggested antenna has an omnidirectional shape for the frequency band from 3.168 GHz to over 15 GHz. There is a two rejection bands, the first one covering 4.87-5.79 GHz with a center frequency of 5.42 GHz, and the other covering 7.2-8.45 GHz with a center frequency of 7.8 GHz. The chosen substrate for the current work is FR-4 having permittivity of 4.3 and thickness of 1.43 mm and the suggested antenna has a small size of 24.5 * 24.5 mm^2. The Experimental results of the manufactured antenna showed agreement with those results of the simulated one. |
|
Open Access | |
A Hybrid Lung Cancer Model for Diagnosis and Stage Classification from Computed Tomography Images | |
Abdalbasit Mohammed Qadir, Peshraw Ahmed Abdalla, and Dana Faiq Abd | |
Pages: 266-274 | |
Version of record online: 12 August 2024 Full Text (PDF) |
|
Detecting pulmonary cancers at early stages is difficult but crucial for patient survival. Therefore, it is essential to develop an intelligent, autonomous, and accurate lung cancer detection system that shows great reliability compared to previous systems and research. In this study, we have developed an innovative lung cancer detection system known as the Hybrid Lung Cancer Stage Classifier and Diagnosis Model (Hybrid-LCSCDM). This system simplifies the complex task of diagnosing lung cancer by categorizing patients into three classes: normal, benign, and malignant, by analyzing computed tomography (CT) scans using a two-part approach: First, feature extraction is conducted using a pre-trained model called VGG-16 for detecting key features in lung CT scans indicative of cancer. Second, these features are then classified using a machine learning technique called XGBoost, which sorts the scans into three categories. A dataset, IQ-OTH/NCCD – Lung Cancer, is used to train and evaluate the proposed model to show its effectiveness. The dataset consists of the three aforementioned classes containing 1190 images. Our suggested strategy achieved an overall accuracy of 98.54 %, while the classification precision among the three classes was 98.63 %. Considering the accuracy, recall, and precision as well as the F1-score evaluation metrics, the results indicated that when using solely computed tomography scans, the proposed (Hybrid-LCSCDM) model outperforms all previously published models. |
|
Open Access | |
Understanding Power Gating Mechanism Based on Workload Classification of Modern Heterogeneous Many-Core Mobile Platform in the Dark Silicon Era | |
Haider Alrudainy, Ali K. Marzook, Muaad Hussein, and Rishad Shafik | |
Pages: 275-283 | |
Version of record online: 18 October 2024 Full Text (PDF) |
|
The rapid progress in mobile computing necessitates energy efficient solutions to support substantially diverse and complex workloads. Heterogeneous many core platforms are progressively being adopted in contemporary embedded implementations for high performance at low power cost estimations. These implementations experience diverse workloads that offer drastic opportunities to improve energy efficiency. In this paper, we propose a novel per core power gating (PCPG) approach based on workload classifications (WLC) for drastic energy cost minimization in the dark silicon era. Core of our paradigm is to use an integrated sleep mode management based on workloads classification indicated by the performance counters. A number of real applications benchmark (PARSEC) are adopted as a practical example of diverse workloads, including memory- and CPU-intensive ones. In this paper, these applications are exercised on Samsung Exynos 5422 heterogeneous many core system showing up to 37% to 110% energy efficient when compared with our most recent published work, and ondemand governor, respectively. Furthermore, we illustrate low-complexity and low-cost runtime per core power gating algorithm that consistently maximize IPS/Watt at all state space. |
|
Open Access | |
Lyapunov Function Control Strategy for a Three-Phase Grid-Tied LCL Filtered Quasi Z-Source Inverter (qZSI) | |
Ali Kadhim Abdulabbas, and Shafaa Mahdi Salih | |
Pages: 284-297 | |
Version of record online: 1 November 2024 Full Text (PDF) |
|
The presented research introduces a control strategy for a three-phase grid-tied LCL-filtered quasi-Z-source inverter (qZSI) using a Lyapunov-function-based method and cascaded proportional-resonant (PR) controllers. The suggested control strategy ensures the overall stability of the closed-loop system and eliminates any steady-state inaccuracy in the grid current. The inverter current and capacitor voltage reference values of qZSI are created by the utilization of cascaded coupled proportional-resonant (PR) controllers. By utilizing synchronous reference frame and Lyapunov function- based control, the requirement to perform derivative operations and anticipate inductance and capacitance are avoided, resulting in achieving the goal of zero steady-state error in the grid current. The qZSI can accomplish shoot-through control by utilizing a simple boost control method. Computer simulations demonstrate that the suggested control strategy effectively achieves the desired control objectives, both in terms of steady-state and dynamic performance. |
|